Funded PhD scholarship
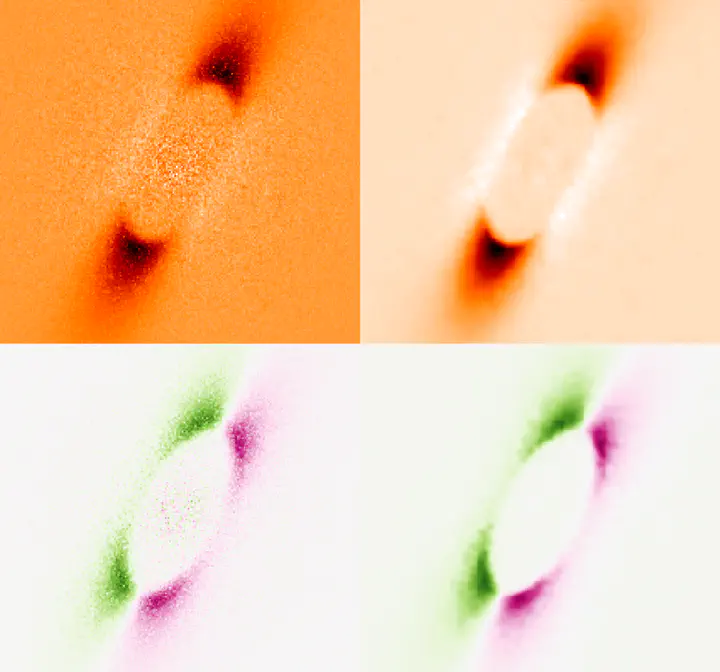
Image reconstruction in high-contrast polarimetric imaging using data driven models
This PhD is funded by PEPR Origins
The PhD involves collaboration between Observatoire de la Côte d’azur (OCA), Ecole Normal Supérieur de Lyon (ENS) and Centre de Recherche Astrophysique de Lyon (CRAL). OCA: André Ferrari (PhD supervisor), Simon Prunet (PhD co-supervisor). ENS: Nelly Pustelnik (co-supervisor), Julian Tachella (co-supervisor). CRAL: Maud Langlois (co-supervisor) and Eric Thiébaut (co-supervisor).
Keywords: Polarimetric imaging, inverse problems, algorithm unfolding, unsupervised learning, plug-and-play algorithms, rotation/scale invariant learning, circumstellar disks imaging
PhD project summary: Polarimetric imaging is an effective technique for high-contrast imaging and characterization of circumstellar environments. The SPHERE IRDIS instrument installed on the VLT in its DPI mode offers the capability to acquire polarimetric images at high contrast and high angular resolution, but dedicated image processing is needed to remove contamination from stellar light, instrumental polarization effects, and instrumental point spread function blurring. In L. Denneulin PhD [1], an observation model for the differential polarimetric imaging mode of SPHERE/IRDIS was derived. A reconstruction strategy (named Rhapsodie) was then proposed to deconvolve the near-infrared polarization signal from circumstellar environments. Rhapsodie relies on variational techniques including weighted data fidelity term, smooth penalization, and additional constraints.
In order to improve the reconstruction performance compared to Rhapsodie, three directions will be explored in this PhD:
-
First, unsupervised hyperparameter selection will be investigated in order to tune the hyperparameters involved in Rhapsodie to make it more easily applicable on real data reconstruction. For this purpose, techniques based on Stein Unbiased Risk Estimator (SURE) will be studied as a first step.
-
Second, Plug&Play Priors (PnP) will be explored in order to provide better regularization and improve reconstruction performance. Such a technique allows us for the alternating minimization of a data-fidelity term to promote data consistency and imposing a learned regularizer in the form of an image denoiser [2]. Additionally, unfolded deep architectures will be developed by unrolling Rhapsodie to design a deep neural network whose parameters are learned either in a supervised or unsupervised way [3,5].
-
Third, the contribution of this work will focus on incorporating rotation and scale equivariance constraints to the priors. The main objective of this task is to optimize both the deep neural network architecture and the parameters estimation step by fine tuning the training set [4,6].
Rhapsodie contains two alternatives: one combining linear direct model with epigraphical constraints and a second one relying on a nonlinear direct model with positivity constraints. If the preliminary studies will focus on linear direct model, its extension to non-linear model including the design of PnP-based approaches in this context will be investigated.
The candidate should hold a Master degree in data processing or astrophysics with a knowledge in machine learning basics. Applicants should send a CV, a cover letter and a transcript of records to André Ferrari.
The successful candidate will join the SI team of J.-L. Lagrange laboratory at OCA which gathers experts in data science, image processing and astrophysics. The successful candidate will also undertake stays in the laboratories of the project’s partners, at Ecole Normal Supérieure de Lyon (ENS) and Centre de Recherche Astrophysique de Lyon (CRAL).
Contacts: André Ferrari andre.ferrari@univ-cotedazur.fr
Deadline for applications: 31st May 2024
Start date: October 2024 or earlier if available.
Contract duration: 3 years
References
- L. Denneulin, M. Langlois, E. Thi'ebaut, and N. Pustelnik, RHAPSODIE : Reconstruction of High- contrAst Polarized SOurces and Deconvolution for cIrcumstellar Environments, A & A, 653, A138 2021.
- U. S. Kamilov, C. A. Bouman, G. T. Buzzard, and B. Wohlberg, Plug-and-play methods for integrating physical and learned models in computational imaging, IEEE Signal Process. Mag., 2022.
- M. Jiu, N. Pustelnik, A deep primal-dual proximal network for image restoration, IEEE JSTSP Special Issue on Deep Learning for Image/Video Restoration and Compression, vol. 15, no. 2,pp. 190–203, Feb. 2021. (PDF).
- J. Tachella, C. Dongdong, and M. Davies. Unsupervised Learning From Incomplete Measurements for Inverse Problems, NeurIPS, 2022.
- M. van den Ende, A. Ferrari, A. Sladen and C. Richard. Deep Deconvolution for Traffic Analysis With Distributed Acoustic Sensing Data. IEEE Transactions on Intelligent Transportation Systems, vol. 24, no. 3, pp. 2947-2962, March 2023.
- M. Weiler and G. Cesa. General E(2)-Equivariant Steerable CNNs. NeurIPS, 2019.